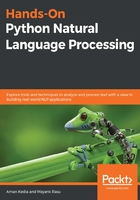
Understanding word normalization
Most of the time, we don't want to have every individual word fragment that we have ever encountered in our vocabulary. We could want this for several reasons, one being the need to correctly distinguish (for example) the phrase U.N. (with characters separated by a period) from UN (without any periods).We can also bring words to their root form in the dictionary. For instance,am,are, andis can be identified by their root form,be. On another front, we can remove inflections from words to bring them down to the same form. Wordscar,cars, andcar'scan all be identified ascar.
Also, common words that occur very frequently and do not convey much meaning, such as the articles a, an, and the, can be removed. However, all these highly depend on the use cases. Wh- words, such as when, why, where, and who, do not carry much information in most contexts and are removed as part of a technique called stopwordremoval, which we will see a little later in the Stopword removal section; however, in situations such as question classification and question answering, these words become very important and should not be removed. Now, with a basic understanding of these techniques, let's deep dive into them in detail.
Stemming
Imagine bringing all of the words computer, computerization, and computerize into one word, compute. What happens here is called stemming. As part of stemming, a crude attempt is made to remove the inflectional forms of a word and bring them to a base form called the stem. The chopped-off pieces are referred to as affixes. In the preceding example, compute is the base form and the affixes are r, rization, and rize, respectively. One thing to keep in mind is that the stem need not be a valid word as we know it. For example, the word traditional would get stemmed to tradit, which is not a valid word in the English dictionary.
The two most common algorithms/methods employed for stemming include the Porter stemmer and the Snowball stemmer. The Porter stemmer supports the English language, whereas the Snowball stemmer, which is an improvement on the Porter stemmer, supports multiple languages, which can be seen in the following code snippet and its output:
from nltk.stem.snowball import SnowballStemmer
print(SnowballStemmer.languages)
Here's the output:
('arabic', 'danish', 'dutch', 'english', 'finnish', 'french', 'german', 'hungarian', 'italian', 'norwegian', 'porter', 'portuguese', 'romanian', 'russian', 'spanish', 'swedish')
One thing to note from the snippet is that the Porter stemmer is one of the offerings provided by the Snowball stemmer. Other stemmers include the Lancaster, Dawson, Krovetz, and Lovins stemmers, among others. We will look at the Porter and Snowball stemmers in detail here.
The Porter stemmer works only with strings, whereas the Snowball stemmer works with both strings and Unicode data. The Snowball stemmer also allows the option to ignore stopwords as an inherent functionality.
Let's now first apply the Porter stemmer to words and see its effects in the following code block:
plurals = ['caresses', 'flies', 'dies', 'mules', 'died', 'agreed', 'owned', 'humbled', 'sized', 'meeting', 'stating',
'siezing', 'itemization', 'traditional', 'reference', 'colonizer', 'plotted', 'having', 'generously']
from nltk.stem.porter import PorterStemmer
stemmer = PorterStemmer()
singles = [stemmer.stem(plural) for plural in plurals]
print(' '.join(singles))
Here's the stemmed output from the Porter stemming algorithm:
caress fli die mule die agre own humbl size meet state siez item tradit refer colon plot have gener
Next, let's see how the Snowball stemmer woulddo on the same text:
stemmer2 = SnowballStemmer(language='english')
singles = [stemmer2.stem(plural) for plural in plurals]
print(' '.join(singles))
Here's the stemmed output of applying the Snowball stemming algorithm:
caress fli die mule die agre own humbl size meet state siez item tradit refer colon plot have generous
As can be seen in the preceding code snippets, the Snowball stemmer requires the specification of a language parameter. In most of cases, its output is similar to that of the Porter stemmer, except for generously, where the Porter stemmer outputs gener and the Snowball stemmer outputs generous. The example shows how the Snowball stemmer makes minor changes to the Porter algorithm, achieving improvements in some cases.
Over-stemming and under-stemming
Potential problems with stemming arise in the form of over-stemming and under-stemming. A situation may arise when words that are stemmed to the same root should have been stemmed to different roots. This problem is referred to as over-stemming. In contrast, another problem occurs when words that should have been stemmed to the same root aren't stemmed to it. This situation is referred to as under-stemming.
Lemmatization
Unlike stemming, wherein a few characters are removed from words using crude methods, lemmatization is a process wherein the context is used to convert a word to its meaningful base form. It helps in grouping together words that have a common base form and so can be identified as a single item. The base form is referred to as the lemma of the word and is also sometimes known as the dictionary form.
Lemmatization algorithms try to identify the lemma form of a word by taking into account the neighborhood context of the word, part-of-speech (POS) tags, the meaning of a word, and so on. The neighborhood can span across words in the vicinity, sentences, or even documents.
Also, the same words can have different lemmas depending on the context. A lemmatizer would try and identify the part-of-speech tags based on the context to identify the appropriate lemma. The most commonly used lemmatizer is the WordNet lemmatizer. Other lemmatizers include the Spacy lemmatizer, TextBlob lemmatizer, and Gensim lemmatizer, and others. In this section, we will explore the WordNet and Spacy lemmatizers.
WordNet lemmatizer
WordNet is a lexical database of English that is freely and publicly available. As part of WordNet, nouns, verbs, adjectives, and adverbs are grouped into sets of cognitive synonyms (synsets), each expressing distinct concepts. These synsets are interlinked using lexical and conceptual semantic relationships. It can be easily downloaded, and the nltk library offers an interface to it that enables you to perform lemmatization.
Let's try and lemmatize the following sentence using the WordNet lemmatizer:
We are putting in efforts to enhance our understanding of Lemmatization
Here is the code:
import nltk
nltk.download('wordnet')
fromnltk.stemimportWordNetLemmatizer
lemmatizer = WordNetLemmatizer()
s = "We are putting in efforts to enhance our understanding of \
Lemmatization"
token_list = s.split()
print("The tokens are: ", token_list)
lemmatized_output = ' '.join([lemmatizer.lemmatize(token) for token \
in token_list])
print("The lemmatized output is: ", lemmatized_output)
Here's the output:
The tokens are: ['We', 'are', 'putting', 'in', 'efforts', 'to', 'enhance', 'our', 'understanding', 'of', 'Lemmatization'] The lemmatized output is: We are putting in effort to enhance our understanding of Lemmatization
As can be seen, the WordNet lemmatizer did not do much here. Out of are, putting, efforts, and understanding, none were converted to their base form.
What are we lacking here?
The WordNet lemmatizer works well if the POS tags are also provided as inputs.
It is really impossible to manually annotate each word with its POS tag in a text corpus. Now, how do we solve this problem and provide the part-of-speech tags for individual words as input to the WordNet lemmatizer?
Fortunately, the nltk library provides a method for finding POS tags for a list of words using an averaged perceptron tagger, the details of which are out of the scope of this chapter.
The POS tags for the sentence We are trying our best to understand Lemmatization here provided by the POS tagging method can be found in the following code snippet:
nltk.download('averaged_perceptron_tagger')
pos_tags = nltk.pos_tag(token_list)
pos_tags
Here's the output:
[('We', 'PRP'), ('are', 'VBP'), ('putting', 'VBG'), ('in', 'IN'), ('efforts', 'NNS'), ('to', 'TO'), ('enhance', 'VB'), ('our', 'PRP$'), ('understanding', 'NN'), ('of', 'IN'), ('Lemmatization', 'NN')]
As can be seen, a list of tuples of the form (the token and POS tag) is returned by the POS tagger. Now, the POS tags need to be converted to a form that can be understood by the WordNet lemmatizer and sent in as input along with the tokens.
The code snippet does what's needed by mapping the POS tags to the first character, which is accepted by the lemmatizer in the appropriate format:
from nltk.corpus import wordnet
##This is a common method which is widely used across the NLP community of practitioners and readers
def get_part_of_speech_tags(token):
"""Maps POS tags to first character lemmatize() accepts.
We are focusing on Verbs, Nouns, Adjectives and Adverbs here."""
tag_dict = {"J": wordnet.ADJ,
"N": wordnet.NOUN,
"V": wordnet.VERB,
"R": wordnet.ADV}
tag = nltk.pos_tag([token])[0][1][0].upper()
return tag_dict.get(tag, wordnet.NOUN)
Now, let’s see how the WordNet lemmatizer performs when the POS tags are also provided as inputs:
lemmatized_output_with_POS_information = [lemmatizer.lemmatize(token, get_part_of_speech_tags(token)) for token in token_list]
print(' '.join(lemmatized_output_with_POS_information))
Here's the output:
We be put in effort to enhance our understand of Lemmatization
The following conversions happened:
- are to be
- putting toput
- effortstoeffort
- understandingtounderstand
Let’s compare this with the Snowball stemmer:
stemmer2 = SnowballStemmer(language='english')
stemmed_sentence = [stemmer2.stem(token) for token in token_list]
print(' '.join(stemmed_sentence))
The following conversions happened:
we are put in effort to enhanc our understand of lemmat
As can be seen, the WordNet lemmatizer makes a sensible and context-aware conversion of the token into its base form, unlike the stemmer, which tries to chop the affixes from the token.
Spacy lemmatizer
The Spacy lemmatizer comes with pretrained models that can parse text and figure out the various properties of the text, such as POS tags, named-entity tags, and so on, with a simple function call. The prebuilt models identify the POS tags and assign a lemma to each token, unlike the WordNet lemmatizer, where the POS tags need to be explicitly provided.
We can install Spacy and download the en model for the English language by running the following command from the command line:
pip install spacy && python -m spacy download en
Now that we have installed spacy, let's see how spacy helps with lemmatization using the following code snippet:
import spacy
nlp = spacy.load('en')
doc = nlp("We are putting in efforts to enhance our understanding of Lemmatization")
" ".join([token.lemma_ for token in doc])
Here's the output:
'-PRON- be put in effort to enhance -PRON- understanding of lemmatization'
The spacy lemmatizer performed a decent job without the input information of the POS tags. The advantage here is that there's no need to look out for external dependencies for fetching POS tags as the information is built into the pretrained model.
Another thing to note in the preceding output is the -PRON- lemma. The lemma for Pronouns is returned as -PRON- in Spacy's default behavior. It can act as a feature or, conversely, can be a limitation, since the exact lemma is not being returned.
Stopword removal
From time to time in the previous sections, a technique called stopword removal was mentioned. We will finally look at the technique in detail here.
What are stopwords?
Stopwords are words such as a, an, the, in, at, and so on that occur frequently in text corpora and do not carry a lot of information in most contexts. These words, in general, are required for the completion of sentences and making them grammatically sound. They are often the most common words in a language and can be filtered out in most NLP tasks, and consequently help in reducing the vocabulary or search space. There is no single list of stopwords that is available universally, and they vary mostly based on use cases; however, a certain list of words is maintained for languages that can be treated as stopwords specific to that language, but they should be modified based on the problem that is being solved.
Let’s look at the stopwords available for English in the nltk library!
nltk.download('stopwords')
from nltk.corpus import stopwords
stop = set(stopwords.words('english'))
", ".join(stop)
Here's the output:
"it's, yours, an, doing, any, mightn't, you, having, wasn't, themselves, just, over, below, needn't, a, this, shan't, them, isn't, was, wouldn't, as, only, his, or, shan, wouldn, don, where, own, were, he, out, do, it, am, won, isn, there, hers, to, ll, most, for, weren, have, by, while, the, re, that, down, haven, has, is, here, itself, all, didn, herself, shouldn, him, ve, who, doesn, m, hadn't, after, further, weren't, at, hadn, should've, too, because, can, now, same, more, she's, wasn, these, yourself, himself, being, very, until, myself, few, so, which, ourselves, they, t, you'd, did, o, aren, but, that'll, such, whom, of, s, you'll, those, doesn't, my, what, aren't, during, hasn, through, will, couldn, i, mustn, needn, mustn't, d, had, me, under, won't, haven't, its, with, when, their, between, if, once, against, before, on, not, you're, each, yourselves, in, and, are, shouldn't, some, nor, her, does, she, off, how, both, our, then, why, again, we, no, y, be, other, ma, from, up, theirs, couldn't, should, into, didn't, ours, about, ain, you've, don't, above, been, than, your, hasn't, mightn"
If you look closely, you'll notice that Wh- words such as who, what, when, why, how, which, where, and whom are part of this list of stopwords; however, in one of the previous sections, it was mentioned that these words are very significant in use cases such as question answering and question classification. Measures should be taken to ensure that these words are not filtered out when the text corpus undergoes stopword removal. Let's learn how this can be achieved by running through the following code block:
wh_words = ['who', 'what', 'when', 'why', 'how', 'which', 'where', 'whom']
stop = set(stopwords.words('english'))
sentence = "how are we putting in efforts to enhance our understanding of Lemmatization"
for word in wh_words:
stop.remove(word)
sentence_after_stopword_removal = [token for token in sentence.split() if token not in stop]
" ".join(sentence_after_stopword_removal)
Here's the output:
'how putting efforts enhance understanding Lemmatization'
The preceding code snippet shows that the sentence how are we putting in efforts to enhance our understanding of Lemmatization gets modified to how putting efforts enhance understanding Lemmatization. The stopwords are, we, in, to, our, and of were removed from the sentence. Stopword removal is generally the first step that is taken after tokenization while building a vocabulary or preprocessing text data.
Case folding
Another strategy that helps with normalization is called case folding. As part of case folding, all the letters in the text corpus are converted to lowercase. The and the will be treated the same in a scenario of case folding, whereas they would be treated differently in a non-case folding scenario. This technique helps systems that deal with information retrieval, such as search engines.
Lamborghini, which is a proper noun, will be treated as lamborghini; whether the user typed Lamborghini or lamborghini would not make a difference, and the same results would be returned.
However, in situations where proper nouns are derived from common noun terms, case folding will become a bottleneck as case-based distinction becomes an important feature here. For instance, General Motors is composed of common noun terms but is itself a proper noun. Performing case folding here might cause issues. Another problem is when acronyms are converted to lowercase. There is a high chance that they will map to common nouns. An example widely used here is CAT which stands for Common Admission Test in India getting converted to cat.
A potential solution to this is to build machine learning models that can use features from a sentence to determine which words or tokens in the sentence should be lowercase and which shouldn't be; however, this approach doesn't always help when users mostly type in lowercase. As a result, lowercasing everything becomes a wise solution.
The language here is a major feature; in some languages, such as English, capitalization from point to point in a text carries a lot of information, whereas in some other languages, cases might not be as important.
The following code snippet shows a very straightforward approach that would convert all letters in a sentence to lowercase, making use of the lower() method available in Python:
s = "We are putting in efforts to enhance our understanding of Lemmatization"
s = s.lower()
s
Here's the output:
'we are putting in efforts to enhance our understanding of lemmatization'
N-grams
Until now, we have focused on tokens of size 1, which means only one word. Sentences generally contain names of people and places and other open compound terms, such as living room and coffee mug. These phrases convey a specific meaning when two or more words are used together. When used individually, they carry a different meaning altogether and the inherent meaning behind the compound terms is somewhat lost. The usage of multiple tokens to represent such inherent meaning can be highly beneficial for the NLP tasks being performed. Even though such occurrences are rare, they still carry a lot of information. Techniques should be employed to make sense of these as well.
In general, these are grouped under the umbrella term of n-grams. When n is equal to 1, these are termed as unigrams. Bigrams, or2-grams, refer to pairs of words, such asdinner table.Phrases such as the United Arab Emiratescomprising three words are termed as trigrams or 3-grams. This naming system can be extended to larger n-grams, but most NLP tasks use only trigrams or lower.
Let’s understand how this works for the following sentence:
Natural Language Processing is the way to go
The phrase Natural Language Processing carries an inherent meaning that would be lost if each of the words in the phrase is processed individually; however, when we use trigrams, these phrases can be extracted together and the meaning gets captured. In general, all NLP tasks make use of unigrams, bigrams, and trigrams together to capture all the information.
The following code illustrates an example of capturing bigrams:
from nltk.util import ngrams
s = "Natural Language Processing is the way to go"
tokens = s.split()
bigrams = list(ngrams(tokens, 2))
[" ".join(token) for token in bigrams]
The output shows the list of bigrams that we captured:
['Natural Language', 'Language Processing', 'Processing is', 'is the', 'the way', 'way to', 'to go']
Let's try and capture trigrams from the same sentence using the following code:
s = "Natural Language Processing is the way to go"
tokens = s.split()
trigrams = list(ngrams(tokens, 3))
[" ".join(token) for token in trigrams]
The output shows the trigrams that were captured from the sentence:
['Natural Language Processing', 'Language Processing is', 'Processing is the', 'is the way', 'the way to', 'way to go']
Taking care of HTML tags
Often, data is scraped from online websites for information retrieval. Since these are mostly HTML pages, there needs to be some preprocessing to remove the HTML tags. HTML tags are mostly noise; however, sometimes they can also carry specific information. Let's think of a use case where a website such as Amazon uses specific tags for identifying features of a product—for example, a <price> tag can be custom created to carry price entries for products. In such scenarios, HTML can be highly useful; however, they are noise for most NLP data.
How do we get rid of them?
BeautifulSoup is an amazing library that helps us with handling such data. The following code snippet shows an example of how this can be achieved:
html = "<!DOCTYPE html><html><body><h1>My First Heading</h1><p>My first paragraph.</p></body></html>"
from bs4 import BeautifulSoup
soup = BeautifulSoup(html)
text = soup.get_text()
print(text)
Here's the output:
My First HeadingMy first paragraph.
How does all this fit into my NLP pipeline?
The steps we discussed should be performed as part of preprocessing the text corpora before applying any algorithms to the data; however, which steps to apply and which to ignore depend on the use case.
These tokens can also be put together after the necessary preprocessing steps that we looked at previously to form the vocabulary. A simple example of this can be seen in the following code:
s = "Natural Language Processing is the way to go"
tokens = set(s.split())
vocabulary = sorted(tokens)
vocabulary
Here's the output:
['Language', 'Natural', 'Processing', 'go', 'is', 'the', 'to', 'way']