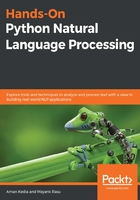
Tokenization
In order to build up a vocabulary, the first thing to do is to break the documents or sentences into chunks called tokens. Each token carries a semantic meaning associated with it. Tokenization is one of the fundamental things to do in any text-processing activity. Tokenization can be thought of as a segmentation technique wherein you are trying to break down larger pieces of text chunks into smaller meaningful ones. Tokens generally comprise words and numbers, but they can be extended to include punctuation marks, symbols, and, at times, understandable emoticons.
Let’s go through a few examples to understand this better:
sentence = "The capital of China is Beijing"
sentence.split()
Here's the output.
['The', 'capital', 'of', 'China', 'is', 'Beijing']
A simple sentence.split() method could provide us with all the different tokens in the sentence The capital of China is Beijing.Each token in the preceding split carries an intrinsic meaning; however, it is not always as straightforward as this.
Issues with tokenization
Consider the sentence and corresponding split in the following example:
sentence = "China's capital is Beijing"
sentence.split()
Here's the output:
["China's", 'capital', 'is', 'Beijing']
In the preceding example, should it be China, Chinas, orChina's? A split method does not often know how to deal with situations containing apostrophes.
In the next two examples, how do we deal with we'll and I'm? We'll indicates we will and I'm indicates I am. What should be the tokenized form of we'll? Should it be well or we'll or we and 'll separately? Similarly, how do we tokenize I'm? An ideal tokenizer should be able to process we'll into two tokens, we and will, and I'm into two tokens, I and am. Let's see how our split method would do in this situation.
Here's the first example:
sentence = "Beijing is where we'll go"
sentence.split()
Here's the output:
['Beijing', 'is', 'where', "we'll", 'go']
Here's the second example:
sentence = "I'm going to travel to Beijing"
sentence.split()
Here's the output:
["I'm", 'going', 'to', 'travel', 'to', 'Beijing']
How do we represent Hong Kong? Should it be two different tokens or should they be one token?
sentence = "Let's travel to Hong Kong from Beijing"
sentence.split()
Here's the output:
["Let's", 'travel', 'to', 'Hong', 'Kong', 'from', 'Beijing']
Here, ideally, Hong Kong should be one token, but think of another sentence: The name of the King is Kong. In such scenarios, Kong should be an individual token. In such situations, context can play a major role in understanding how to treat similar token representations when the context varies. Tokens of size 1, such asKong, are referred to as unigrams, whereas tokens of size 2, such asHong Kong, are referred to asbigrams. These can be generalized under the wing ofn-grams, which we'll discuss towards the end of this chapter.
How do we deal with periods? How do we understand whether they signify the end of a sentence or indicate an abbreviation?
In the following code snippet and subsequent output, the period between M and S is actually indicative of an abbreviation:
sentence = "A friend is pursuing his M.S from Beijing"
sentence.split()
Here's the output:
['A', 'friend', 'is', 'pursuing', 'his', 'M.S', 'from', 'Beijing']
In the next example, does a token such as ummcarry any meaning? Shouldn't it be removed? Even though a token such asummis not a part of English vocabulary, it becomes important in use cases where speech synthesis is involved as it indicates that the person is taking a pause here and trying to think of something. Again, as well as the context, the notion of the use case also matters when understanding where something should be tokenized or simply removed as a fragment of text that doesn't convey any meaning:
sentence = "Most of the times umm I travel"
sentence.split()
The rise of social media platforms has resulted in a massive influx of user data, which is a rich mine of information to understand individuals and communities; however, it has also catered to the rise of a world of emoticons, short forms, new abbreviations (often called the millennial language), and so on. There is a need to understand this ever-growing kind of text, as well those cases where, for instance, a characterP used with a colon (:) and hyphen (-) denotes a face with a stuck -out tongue. Hashtags are another very common thing on social media that are mostly indicative of summaries or emotions behind a Facebook post or a tweet on Twitter. An example of this is shown in the following example. Such growth leads to the development of tokenizers such as TweetTokenizer:
sentence = "Beijing is a cool place!!! :-P <3 #Awesome"
sentence.split()
Here's the output:
['Beijing', 'is', 'a', 'cool', 'place!!!', ':-P', '<3', '#Awesome']
In the next section, we will look at TweetTokenizer and a few other standard tokenizers available from the nltk library.
Different types of tokenizers
Based on the understanding we have developed so far, let's discuss the different types of tokenizers that are readily available for usage and see how these could be leveraged for the proper tokenization of text.
Regular expressions
Regular expressions are sequences of characters that define a search pattern. They are one of the earliest and are still one of the most effective tools for identifying patterns in text. Imagine searching for email IDs in a corpus of text. These follow the same pattern and are guided by a set of rules, no matter which domain they are hosted upon. Regular expressions are the way to go for identifying such things in text data instead of trying out machine learning-oriented techniques. Other notable examples where regular expressions have been widely employed include the SUTime offering from Stanford NLP, wherein tokenization based on regular expressionsis used to identify the date, time, duration, and set type entities in text. Look at the following sentence:
Last summer, they met every Tuesday afternoon, from 1:00 pm to 3:00 pm.
For this sentence, the SUTime library would return TIMEX expressions where each TIMEX expression would indicate the existence of one of the aforementioned entities:
Last summer, they met every Tuesday afternoon, from 1:00 pm to 3:00 pm.

The TIMEX expressions can be parsed to convert them into a user-readable format.
Try it out!
Regular expressions-based tokenizers
The nltk package in Python provides a regular expressions-based tokenizers (RegexpTokenizer) functionality. It can be used to tokenize or split a sentence based on a provided regular expression. Take the following sentence:
A Rolex watch costs in the range of $3000.0 - $8000.0 in the USA.
Here, we would like to have expressions indicating money, alphabetic sequences, and abbreviations together. We can define a regular expression to do this and pass the utterance to the corresponding tokenizer object, as shown in the following code block:
from nltk.tokenize import RegexpTokenizer
s = "A Rolex watch costs in the range of $3000.0 - $8000.0 in USA."
tokenizer = RegexpTokenizer('\w+|\$[\d\.]+|\S+')
tokenizer.tokenize(s)
Here's the output:
['A', 'Rolex', 'watch', 'costs', 'in', 'the', 'range', 'of', '$3000.0', '-', '$8000.0', 'in', 'USA', '.']
Now, how did this work?
The \w+|\$[\d\.]+|\S+regular expression allows three alternative patterns:
- First alternative: \w+ that matches any word character (equal to [a-zA-Z0-9_]). The +is a quantifier and matches between one and unlimited times as many times as possible.
- Second alternative: \$[\d\.]+. Here, \$matches the character $, \dmatches a digit between 0 and 9, \. matches the character . (period), and +again acts as a quantifier matching between one and unlimited times.
- Third alternative: \S+. Here, \Saccepts any non-whitespace character and +again acts the same way as in the preceding two alternatives.
There are other tokenizers built on top of the RegexpTokenizer, such as the BlankLine tokenizer, which tokenizes a string treating blank lines as delimiters where blank lines are those that contain no characters except spaces or tabs.
The WordPunct tokenizer is another implementation on top of RegexpTokenizer, which tokenizes a text into a sequence of alphabetic and nonalphabetic characters using the regular expression \w+|[^\w\s]+.
Try it out!
Build a regular expression to figure out email IDs from the text. Validate your expression at https://regex101.com.
Treebank tokenizer
The Treebank tokenizer also uses regular expressions to tokenize text according to the Penn Treebank (https://catalog.ldc.upenn.edu/docs/LDC95T7/cl93.html). Here, words are mostly split based on punctuation.
The Treebank tokenizer does a great job of splitting contractions such as doesn't to does and n't. It further identifies periods at the ends of lines and eliminates them. Punctuation such as commas is split if followed by whitespaces.
Let’s look at the following sentence and tokenize it using the Treebank tokenizer:
I'm going to buy a Rolex watch that doesn't cost more than $3000.0
The code is as follows:
from nltk.tokenize import TreebankWordTokenizer
s = "I'm going to buy a Rolex watch that doesn't cost more than $3000.0"
tokenizer = TreebankWordTokenizer()
tokenizer.tokenize(s)
Here's the output:
['I', "'m", 'going', 'to', 'buy', 'a', 'Rolex', 'watch', 'which', 'does', "n't", 'cost', 'more', 'than', '$', '3000.0']
As can be seen in the example and corresponding output, this tokenizer primarily helps in analyzing each component in the text separately. The I'm gets split into two components, namely the I, which corresponds to a noun phrase, and the 'm, which corresponds to a verb component. This split allows us to work on individual tokens that carry significant information that would have been difficult to analyze and parse if it was a single token. Similarly, doesn't gets split into does and n't, helping to better parse and understand the inherent semantics associated with the n't, which indicates negation.
TweetTokenizer
As discussed earlier, the rise of social media has given rise to an informal language wherein people tag each other using their social media handles and use a lot of emoticons, hashtags, and abbreviated text to express themselves. We need tokenizers in place that can parse such text and make things more understandable. TweetTokenizer caters to this use case significantly. Let's look at the following sentence/tweet:
@amankedia I'm going to buy a Rolexxxxxxxx watch!!! :-D #happiness #rolex <3
The tweet contains a social media handle, amankedia, a couple of hashtags in the form of #happiness and #rolex, and :-D and <3 emoticons. The next code snippet and the corresponding output show how all the text gets tokenized using TweetTokenizer to take care of all of these occurrences.
Consider the following example:
from nltk.tokenize import TweetTokenizer
s = "@amankedia I'm going to buy a Rolexxxxxxxx watch!!! :-D #happiness #rolex <3"
tokenizer = TweetTokenizer()
tokenizer.tokenize(s)
Here's the output:
['@amankedia', "I'm", 'going', 'to', 'buy', 'a', 'Rolexxxxxxxx', 'watch', '!', '!', '!', ':-D', '#happiness', '#rolex', '<3']
Another common thing with social media writing is the use of expressions such as Rolexxxxxxxx. Here, a lot of x's are present in addition to the normal one; it is a very common trend and should be addressed to bring it to a form as close to normal as possible.
The TweetTokenizer provides two additional parameters in the form of reduce_len, which tries to reduce the excessive characters in a token. The word Rolexxxxxxxx is actually tokenized as Rolexxx in an attempt to reduce the number of x's present:
from nltk.tokenize import TweetTokenizer
s = "@amankedia I'm going to buy a Rolexxxxxxxx watch!!! :-D #happiness #rolex <3"
tokenizer = TweetTokenizer(strip_handles=True, reduce_len=True)
tokenizer.tokenize(s)
Here's the output:
["I'm", 'going', 'to', 'buy', 'a', 'Rolexxx', 'watch', '!', '!', '!', ':-D', '#happiness', '#rolex', '<3']
The parameter strip_handles, when set to True, removes the handles mentioned in a post/tweet. As can be seen in the preceding output, @amankedia is stripped, since it is a handle.
One more parameter that is available with TweetTokenizer is preserve_case, which, when set to False, converts everything to lower case in order to normalize the vocabulary. The default value for this parameter is True.