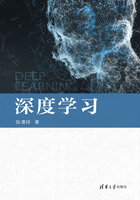
参考文献
[1] Legendre A M. Nouvelles Méethodes Pour la Déetermination des Orbites des Comèetes[M]. Paries: Courcier, 1805.
[2] Carl F G. Theoria Motus Corporum Coelestium in Sectionibus Conicis Solem Ambientum[M]. United Kingdom: Cambridge University Press, 1809.
[3] Carl F G. Theoria Combinationis Observationum Erroribus Minimis Obnoxiae[M]. Carolina: Nabu Press, 1821.
[4] Pearson K. On the criterion that a given system of deviations from the probable in the case of a correlated system of variables is such that it can be reasonably supposed to have arisen from random sampling[J]. Philosophical Magazine, 1900, 50(302): 157–175.
[5] Pearson K. On lines and planes of closest fit to systems of points in space[J]. Philosophical Magazine, 1901, 2(11): 559–572.
[6] Cochran W G. The Chi-square test of goodness of fit[J]. The Annals of Mathematical Statistics, 1952, 23: 315–345.
[7] Student(Gosset W S). The probable error of a mean[J]. Biometrika, 1908, 6(1): 1–25.
[8] Fisher R A. On the mathematical foundations of theoretical statistics[J]. Philosophical Transactions of the Royal Society A, 1922, 222: 309–368.
[9] Aldrich J. Fisher and the making of maximum likelihood 1912–1922[J]. Statistical Science, 1997, 12(3): 162–176.
[10] Fisher R A. The correlation between relatives on the supposition of Mendelian inheritance[J]. Philosophical Transactions of the Royal Society of Edinburgh, 1918, 52: 399–433.
[11] Neyman J. Outline of a theory of statistical estimation based on the classical theory of probability[J]. Philosophical Transactions of the Royal Society A, 1937, 236(767): 333–380.
[12] Wishart J. The generalised product moment distribution in samples from a normal multivariate population[J]. Biometrika, 1928, 20A(1–2): 32–52.
[13] Lehmann E L. Hsu’s work on inference[J]. The Annals of Statistics, 1979, 7(3): 471–473.
[14]Samuel A L. Some studies in machine learning using the game of checkers[J]. IBM Journal of Research and Development, 1959, 3(3): 210–229.
[15] Rosenblatt F. The Perceptron–a perceiving and recognizing automaton: 85-460-1[R]. Cornell Aeronautical Laboratory, 1957.
[16] Rosenblatt F. The perceptron: a probabilistic model for information storage and organization in the brain[J]. Psychological Review, 1958, 65(6): 386–408.
[17] Minsky M, Papert S. Perceptrons: An Introduction to Computational Geometry[M]. Cambridge, MA: The MIT Press, 1969.
[18] Linnainmaa S. The representation of the cumulative rounding error of an algorithm as a Taylor expansion of the local rounding errors[D]. Helsinki: University of Helsinki, 1970.
[19] Werbos P. Beyond regression: new tools for prediction and analysis in the behavioral sciences[D]. Boston: Harvard University, 1974.
[20] Werbos P. Backpropagation through time: what it does and how to do it[C]. Proceedings of the IEEE, 1990, 78(10): 1550–1560.
[21] Rumelhart D E, Hinton G E, Williams R J. Learning representations by backpropagating errors[J]. Nature, 1986, 323(6088): 533–536.
[22] Hubel D H, Wiesel T N. Receptive fields of single neurones in the cat’s striate cortex[J]. The Journal of Physiology, 1959, 124(3): 574–591.
[23] Hubel D H, Wiesel T N. Receptive fields, binocular interaction and functional architecture in the cat’s visual cortex[J]. The Journal of Physiology, 1962, 160(1): 106–154.
[24] Fukushima K. Neocognitron: a self-organizing neural network model for a mechanism of pattern recognition unaffected by shift in position[J]. Biological Cybernetics, 1980, 36(4): 193–202.
[25] Fukushima K, Miyake S, Ito T. Neocognitron: a neural network model for a mechanism of visual pattern recognition[J]. IEEE Transactions on Systems, Man, and Cybernetics, 1983, SMC-13(5): 826–834.
[26] LeCun Y, Boser B, Denker J S, et al. Backpropagation applied to handwritten zip code recognition[J]. Neural Computation, 1989, 1(4): 541–551.
[27] Cun Y L, Denker J S, Solla S A. Optimal brain damage[C]. The Proceedings of International Conference on Neural Information Processing Systems. Denver, Colorado, USA, November 27–30, 1989.
[28] LeCun Y, Bottou L, Bengio Y, et al. Gradient-Based Learning Applied to Document Recognition[J]. Proceedings of IEEE, 1998, 86(11): 2278–2324.
[29] Hochreiter S. Untersuchungen zu dynamischen neuronalen Netzen[D]. Munich: Institut of Informatik, Technische University, 1991.
[30] Hochreiter S, Bengio Y, Frasconi P, et al. Gradient Flow in Recurrent Nets: The Difficulty of Learning Long-Term Dependencies[M]. Piscataway: IEEE Press, 2001.
[31] Boser B E, Guyon I M, Vapnik V. A training algorithm for optimal margin classifiers[C]. Proceedings of the Fifth Annual Workshop on Computational Learning Theory, Pittsburgh, PA, USA. July 27–29, 1992: 144–152.
[32] Guyon I, Boser B E, Vapnik V. Automatic capacity tuning of very large VC-dimension classifiers[J]. Advances in Neural Information Processing Systems, 1993, 5: 147–155.
[33] Cortes C, Vapnik V. Support-vector networks[J]. Machine Learning, 1995, 20(3): 273–297.
[34] Hinton G E, Salakhatdinov R R. Reducing the dimensionality of data with neural networks[J]. Science, 2006, 313(5786): 504–507.
[35] Hinton G E, Osindero S, Teh Y W. A fast learning algorithm for deep belief nets[J]. Neural Computation, 2006, 18(7): 1527–1554.
[36] Krizhevsky A, Sutskever I, Hinton G E. ImageNet classification with deep convolutional neural networks[C]. Proceedings of International Conference on Neural Information Processing Systems. Lake Tahoe, Nevada, United States. December 3-6, 2012: 1106–1114.
[37] Russakovsky O, Deng J, Su H, et al. ImageNet large scale visual recognition challenge[J]. arxiv, 2014, 1409: 0575.
[38] Graves A, Mohamed A, Hinton G. Speech recognition with deep recurrent neural networks[C]. Proceedings of IEEE international conference on Acoustics, speech and signal processing. Vancouver, British Columbia, Canada. May 26-31, 2013: 6645–6649.
[39] Chen Aixiang, Chen Bingchuan, Chai Xiaolong, et al. A novel stochastic stratified average gradient method: convergence rate and its complexity[C]. Proceedings of International Joint Conference of Neural Networks. Brazil. Rio de Janeiro, July 08, 2018, arXiv: 1710. 07783.
[40] Goodfellow I, Bengio Y, Courville A. Deep Learning[M]. Cambridge, MA: The MIT Press, 2016.
[41] Aizerman M A, Braverman E M, Rozonoer L I. Theoretical foundations of the potential function method in pattern recognition learning[J]. Automation and Remote Control, 1964, 25: 821–837.
[42] Mercer J. Functions of positive and negative type, and their connection with the theory of integral equations[J]. Philosophical Transactions of the Royal Society A: Mathematical, Physical and Engineering Sciences, 1909, 209: 415–446.
[43] Mercer J. Functions of positive and negative type, and their connection with the theory of integral equations[C]. Proceedings of the Royal Society Serial A, London. 1908(559), 83: 69–70.
[44] Cox D R. The regression analysis of binary sequences(with discussion)[J]. Journal of the Royal Statistical Society, Series B(Methodological), 1958, 20(2): 215–242.
(1) 为简便起见,该数据集来自斯坦福大学华裔教授吴恩达的机器学习公开课。
(2) 本章使用前一种形式,其等价的后一种形式将在后续神经网络或深度模型中使用。
(3) 这个概念来自线性代数,n个无关组可构成一个n维空间。
(4) 误差可分解成方差和偏倚两项之和,即e2=σ2+B2,其中方差过大引起的误差可通过加大训练样本来减小。但偏倚主要是模型不恰当引起的,如果误差过大主要是因为偏倚,则无论怎么加大样本都不能有效减少误差。
(5) 这个变换函数的反函数被称为连接函数,后面的广义线性模型会对此做进一步解释。
(6) 由于广义线性模型和一般线性模型首字母均是GLM,本书约定复数形式的GLMs为广义线性模型的缩写,单数形式的GLM为一般线性模型的缩写。
(7) 更一般的形式应该是线性预测部分、连接函数和方差函数(描述方差如何依赖于均值)三部分组成,本书不考虑方差随均值变化的情况。