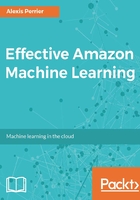
Adding features?
In general, adding new features that are correlated in some ways to the outcome brings information and improves a model. However, adding too many features with little predictive power may end up bringing confusion to that same model and in the end degrading its performance.
Feature selection by removal of the least interesting features is worth trying when the sample size is small compared to the number of features; it leads to too few observations or too many features. There are different strategies (http://machinelearningmastery.com/an-introduction-to-feature-selection/) to identify and remove weak features. Selecting features based on their correlation with the outcome and discarding features with little or no correlation with the outcome will usually improve your model.