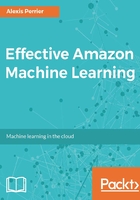
Understanding predictive analytics
Data Science, predictive analytics, machine learning -- these terms are used in many ways and sometimes overlap each other. What they actually refer to is not always obvious.
Data science is one of the most popular technical domains whose trend erupted after the publication of the often cited Harvard Business Review article of October 2012, Data Scientist: The Sexiest Job of the 21st Century (https://hbr.org/2012/10/data-scientist-the-sexiest-job-of-the-21st-century). Data science can be seen as an evolution from data mining and data analytics. Data mining is about exploring data to discover patterns that potentially lead to decisions and actions at the business level. Data science englobes data analytics and regroups a wider scope of domains, such as statistics, data visualization, predictive analytics, software engineering, and so on, under one very large umbrella.
Predictive analytics is the art of predicting future events based on past observations. It requires your data to be organized in a certain way with predictor variables and outcomes well identified. As the Danish politician Karl Kristian Steincke once said, "Making predictions is difficult especially about the future." (This quote has also been attributed to Niels Bohr, Yogi Berra and others by http://quoteinvestigator.com/2013/10/20/no-predict/). Predictive analytics applications are perse and far ranging: predicting consumer behavior, natural events (weather, earthquakes, and so on), people's behavior or health, financial markets, industrial applications, and so on. Predictive analytics relies on supervised learning, where data and labels are given to train the model.
Machine learning comprises the tools, methods, and concepts for computers to optimize models used for predictive analytics or other goals.
Machine learning's scope is much larger than predictive analytics. Three different types of machine learning are usually considered:
- Supervised learning: Assumes that a certain amount of training data with known outcomes is available and can be used to train the model. Predictive analytics is part of supervised learning.
- Unsupervised learning: Is about finding patterns in existing data without knowing the outcome. Clustering customer behavior or reducing the dimensions of the dataset for visualization purposes are examples of unsupervised learning.
- Reinforcement learning: Is the third type of machine learning, where agents learn to act on their own when given a set of rules and a specific reward schema. Examples of reinforcement learning applications include AlphaGo, Google's world championship Go algorithm, self-driving cars, and semi-autonomous robots. AlphaGo learned from thousands of past games and was able to beat the world Go champion in March 2016 (https://www.wired.com/2016/03/go-grandmaster-lee-sedol-grabs-consolation-win-googles-ai/). A classic reinforcement learning implementation follows this schema, where an agent adapts its actions on an environment based on the resulting rewards:

The difference between supervised and unsupervised learning in the context of binary classification and clustering is illustrated in the following two figures:
- For supervised learning, the original dataset is composed of two classes (squares and circles), and we know from the start to which class each sample belongs. Giving that information to a binary classification algorithm allows for a somewhat optimized separation of the two classes. Once that separating frontier is known, the model (the line) can be used to predict the class of new samples depending on which side the sample ends up being:

- In unsupervised learning, the different classes are not known. There is no ground truth. The data is given to an algorithm along with some parameters, such as the number of classes to be found, and the algorithm finds the best set of clusters in the original dataset according to a defined criteria or metric. The results may be very dependent on the initialization parameters. There is no truth, no accuracy, just an interpretation of the data. The following figure shows the results obtained by a clustering algorithm asked to find three classes in the original data:

The reader will notice at this point that the book is titled Amazon Machine Learning and not Amazon Predictive Analytics. This is a bit misleading, as machine learning covers many applications and problems besides predictive analytics. However, calling the service machine learning leaves the door open for Amazon to roll out future services that are not focused on predictive analytics. The following figure maps out the relationships between data science terms:
