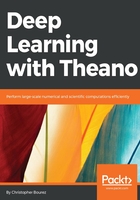
Optimization and other update rules
Learning rate is a very important parameter to set correctly. Too low a learning rate will make it difficult to learn and will train slower, while too high a learning rate will increase sensitivity to outlier values, increase the amount of noise in the data, train too fast to learn generalization, and get stuck in local minima:

When training loss does not improve anymore for one or a few more iterations, the learning rate can be reduced by a factor:

It helps the network learn fine-grained differences in the data, as shown when training residual networks (Chapter 7, Classifying Images with Residual Networks):

To check the training process, it is usual to print the norm of the parameters, the gradients, and the updates, as well as NaN values.
The update rule seen in this chapter is the simplest form of update, known as Stochastic Gradient Descent (SGD). It is a good practice to clip the norm to avoid saturation and NaN values. The updates list given to the theano
function becomes this:
def clip_norms(gs, c): norm = T.sqrt(sum([T.sum(g**2) for g in gs])) return [ T.switch(T.ge(norm, c), g*c/norm, g) for g in gs] updates = [] grads = T.grad(cost, params) grads = clip_norms(grads, 50) for p,g in zip(params,grads): updated_p = p - learning_rate * g updates.append((p, updated_p))
Some very simple variants have been experimented with in order to improve the descent, and are proposed in many deep learning libraries. Let's see them in Theano.
Momentum
For each parameter, a momentum (v, as velocity) is computed from the gradients accumulated over the iterations with a time decay. The previous momentum value is multiplied by a decay parameter between 0.5 and 0.9 (to be cross-validated) and added to the current gradient to provide the new momentum value.
The momentum of the gradients plays the role of a moment of inertia in the updates, in order to learn faster. The idea is also that oscillations in successive gradients will be canceled in the momentum, to move the parameter in a more direct path towards the solution:

The decay parameter between 0.5 and 0.9 is a hyperparameter usually referred to as the momentum, in an abuse of language:
updates = [] grads = T.grad(cost, params) grads = clip_norms(grads, 50) for p,g in zip(params,grads): m = theano.shared(p.get_value() * 0.) v = (momentum * m) - (learning_rate * g) updates.append((m, v)) updates.append((p, p + v))
Nesterov Accelerated Gradient
Instead of adding v to the parameter, the idea is to add directory the future value of the momentum momentum v - learning_rate g
, in order to have it compute the gradients in the next iteration directly at the next position:
updates = [] grads = T.grad(cost, params) grads = clip_norms(grads, 50) for p, g in zip(params, grads): m = theano.shared(p.get_value() * 0.) v = (momentum * m) - (learning_rate * g) updates.append((m,v)) updates.append((p, p + momentum * v - learning_rate * g))
Adagrad
This update rule, as well as the following rules consists of adapting the learning rate parameter-wise (differently for each parameter). The element-wise sum of squares of the gradients is accumulated into a shared variable for each parameter in order to decay the learning rate in an element-wise fashion:
updates = [] grads = T.grad(cost, params) grads = clip_norms(grads, 50) for p,g in zip(params,grads): acc = theano.shared(p.get_value() * 0.) acc_t = acc + g ** 2 updates.append((acc, acc_t)) p_t = p - (learning_rate / T.sqrt(acc_t + 1e-6)) * g updates.append((p, p_t))
Adagrad
is an aggressive method, and the next two rules, AdaDelta
and RMSProp
, try to reduce its aggression.
AdaDelta
Two accumulators are created per parameter to accumulate the squared gradients and the updates in moving averages, parameterized by the decay rho
:
updates = [] grads = T.grad(cost, params) grads = clip_norms(grads, 50) for p,g in zip(params,grads): acc = theano.shared(p.get_value() * 0.) acc_delta = theano.shared(p.get_value() * 0.) acc_new = rho * acc + (1 - rho) * g ** 2 updates.append((acc,acc_new)) update = g * T.sqrt(acc_delta + 1e-6) / T.sqrt(acc_new + 1e-6) updates.append((p, p - learning_rate * update)) updates.append((acc_delta, rho * acc_delta + (1 - rho) * update ** 2))
RMSProp
This updates rule is very effective in many cases. It is an improvement of the Adagrad
update rule, using a moving average (parameterized by rho
) to get a less aggressive decay:
updates = [] grads = T.grad(cost, params) grads = clip_norms(grads, 50) for p,g in zip(params,grads): acc = theano.shared(p.get_value() * 0.) acc_new = rho * acc + (1 - rho) * g ** 2 updates.append((acc, acc_new)) updated_p = p - learning_rate * (g / T.sqrt(acc_new + 1e-6)) updates.append((p, updated_p))
Adam
This is RMSProp
with momemtum, one of the best choices for the learning rule. The time step is kept track of in a shared variable, t
. Two moving averages are computed, one for the past squared gradients, and the other for past gradient:
b1=0.9, b2=0.999, l=1-1e-8 updates = [] grads = T.grad(cost, params) grads = clip_norms(grads, 50) t = theano.shared(floatX(1.)) b1_t = b1 * l **(t-1) for p, g in zip(params, grads): m = theano.shared(p.get_value() * 0.) v = theano.shared(p.get_value() * 0.) m_t = b1_t * m + (1 - b1_t) * g v_t = b2 * v + (1 - b2) * g**2 updates.append((m, m_t)) updates.append((v, v_t)) updates.append((p, p - (learning_rate * m_t / (1 - b1**t)) / (T.sqrt(v_t / (1 - b2**t)) + 1e-6)) ) updates.append((t, t + 1.))
To conclude on update rules, many recent research papers still prefer the simple SGD rule, and work the architecture and the initialization of the layers with the correct learning rate. For more complex networks, or if the data is sparse, the adaptive learning rate methods are better, sparing you the pain of finding the right learning rate.