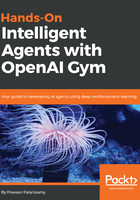
Installing tools and libraries needed for deep reinforcement learning
Chapter 2, Reinforcement Learning and Deep Reinforcement Learning, prepped you with the basics of reinforcement learning. With that theoretical background, we will be able to implement some cool algorithms. Before that, we will make sure we have the required tools and libraries at our disposal.
We can actually write cool reinforcement learning algorithms in Python without using any higher-level libraries. However, when we start to use function approximators for the value functions or the policy, and especially if we use deep neural networks as the function approximators, it is better to use highly optimized deep learning libraries instead of writing our own routines. A deep learning library is the major tool/library that we will need to install. There are different libraries out there today: PyTorch, TensorFlow, Caffe, Chainer, MxNet, and CNTK, to name a few. Each library has its own philosophy, merits, and demerits, depending on the use cases. We will be using PyTorch for developing the deep reinforcement learning algorithms in this book, due to its simplicity of use and dynamic graph definition. The algorithms we will discuss and the way we approach the implementation in this book will be explained in such a way that you can easily re-implement them using the framework of your choice.
If you do not have a GPU on your machine, or if you do not plan to use your GPU for training, you may skip the GPU driver installation steps and can install a CPU-only binary version of PyTorch using the following conda command:
(rl_gym_book) praveen@ubuntu:~$ conda install pytorch-cpu torchvision -c pytorch
Note that you will not be able to accelerate the training of some of the agents we will develop as part of this book, which can utilize a GPU for faster training.