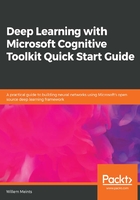
Preface
Artificial intelligence (AI) is here to enhance humans by automating some of the tasks we do every day, so we can spend more time fully realizing our potential. We've been using software programs as tools to automate many of the simpler tasks. Now it is time to take on the challenge of automating more complicated tasks.
There's a lot happening in the area of AI and more people than ever are looking to expand their existing toolkit with new techniques to make their software smarter. Machine learning, and especially deep learning, are highly important tools with which we can enhance what we are already doing with our computers.
This book aims to help you get to grips with one of the most popular deep learning tools, CNTK. We will look at what this relatively young open source deep learning framework offers. At the end of this book, you'll have a solid understanding of the framework and some of the scenarios in which it can be used.
What this book covers
Chapter 1, Getting Started with CNTK, introduces you to the CNTK framework and the world of deep learning. It explains how to install the tools on your computer and how to use a GPU with CNTK.
Chapter 2, Building Neural Networks with CNTK, explains how to build your first neural network with CNTK. We dive into the basic building blocks and see how to train a neural network with CNTK.
Chapter 3, Getting Data into Your Neural Network, shows you different methods of loading data for training neural networks. You'll learn how to work with both small datasets, and datasets that don't fit in your computer's memory.
Chapter 4, Validating Model Performance, teaches you how to work with metrics to validate the performance of your neural network. You'll learn how to validate regression models and classification models and what to look for when trying to debug your neural network.
Chapter 5, Working with Images, explains how to use convolutional neural networks to classify images. We'll show you the building blocks needed to work with spatially-ordered data. We'll also show you some of the most well-known neural network architectures for working with images.
Chapter 6, Working with Time Series Data, teaches you how to use recurrent neural networks to build models that can reason over time. We'll explain the various building blocks that you need to build and validate a recurrent neural network yourself, based on a IoT sample.
Chapter 7, Deploying Models to Production, shows you what it takes to deploy deep learning models to production. We'll take a look at a DevOps environment with a continuous integration/continuous deployment (CI/CD) pipeline to teach you what it takes to train and deploy models in an agile engineering environment. We'll show you how you can use a tool such as Azure Machine Learning service to take your machine learning efforts to the next level.