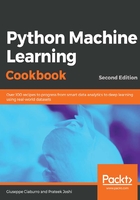
上QQ阅读APP看书,第一时间看更新
How to do it...
Let's see how to group data using agglomerative clustering:
- The full code for this recipe is given in the agglomerative.py file that's provided to you. Now let's look at how it's built. Create a new Python file, and import the necessary packages:
import numpy as np import matplotlib.pyplot as plt from sklearn.cluster import AgglomerativeClustering from sklearn.neighbors import kneighbors_graph
- Let's define the function that we need to perform agglomerative clustering:
def perform_clustering(X, connectivity, title, num_clusters=3, linkage='ward'): plt.figure() model = AgglomerativeClustering(linkage=linkage, connectivity=connectivity, n_clusters=num_clusters) model.fit(X)
- Let's extract the labels and specify the shapes of the markers for the graph:
# extract labels labels = model.labels_ ` # specify marker shapes for different clusters markers = '.vx'
- Iterate through the datapoints and plot them accordingly using different markers:
for i, marker in zip(range(num_clusters), markers): # plot the points belong to the current cluster plt.scatter(X[labels==i, 0], X[labels==i, 1], s=50, marker=marker, color='k', facecolors='none') plt.title(title)
- In order to demonstrate the advantage of agglomerative clustering, we need to run it on datapoints that are linked spatially, but also located close to each other in space. We want the linked datapoints to belong to the same cluster, as opposed to datapoints that are just spatially close to each other. Let's, now define a function to get a set of datapoints on a spiral:
def get_spiral(t, noise_amplitude=0.5): r = t x = r * np.cos(t) y = r * np.sin(t) return add_noise(x, y, noise_amplitude)
- In the previous function, we added some noise to the curve because it adds some uncertainty. Let's define this function:
def add_noise(x, y, amplitude): X = np.concatenate((x, y)) X += amplitude * np.random.randn(2, X.shape[1]) return X.T
- Now let's define another function to get datapoints located on a rose curve:
def get_rose(t, noise_amplitude=0.02): # Equation for "rose" (or rhodonea curve); if k is odd, then # the curve will have k petals, else it will have 2k petals k = 5 r = np.cos(k*t) + 0.25 x = r * np.cos(t) y = r * np.sin(t) return add_noise(x, y, noise_amplitude)
- Just to add more variety, let's also define a hypotrochoid function:
def get_hypotrochoid(t, noise_amplitude=0): a, b, h = 10.0, 2.0, 4.0 x = (a - b) * np.cos(t) + h * np.cos((a - b) / b * t) y = (a - b) * np.sin(t) - h * np.sin((a - b) / b * t) return add_noise(x, y, 0)
- We are now ready to define the main function:
if __name__=='__main__': # Generate sample data n_samples = 500 np.random.seed(2) t = 2.5 * np.pi * (1 + 2 * np.random.rand(1, n_samples)) X = get_spiral(t) # No connectivity connectivity = None perform_clustering(X, connectivity, 'No connectivity') # Create K-Neighbors graph connectivity = kneighbors_graph(X, 10, include_self=False) perform_clustering(X, connectivity, 'K-Neighbors connectivity') plt.show()
If you run this code, you will get the following output if we don't use any connectivity:

The second output diagram looks like the following:

As you can see, using the connectivity feature enables us to group the datapoints that are linked to each other as opposed to clustering them, based on their spatial locations.