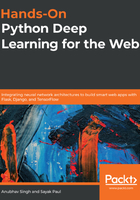
What is DL?
Now comes the most exciting part and probably the hottest technical term of this century. Reality apart, we now understand the learning to some extent, so let's get to the first part of the term deep learning—deep.
DL is a type of machine learning but it is purely based on neural networks. We will take a look at neural networks too but in the next chapter. The basic objective of any machine learning system is to learn useful representations of the data given to it. But what makes DL different? It turns out that DL systems treat data as a representation of layers. For example, an image can be treated as a representation of layers of varying properties such as edges, contours, orientation, texture, and gradients. The following diagram from the book, Deep Learning with Python, by Fran?ois Chollet captures this idea nicely:
In the preceding diagram, a DL system is being employed to classify an image of a hand-written digit. The system takes the image of the handwritten digit as its input and tries to learn its underlying representations. In the first layer, the system learns generic features such as strokes and lines. As the layers increase, it learns about the features that are more specific to the given image. The more the number of layers, the deeper the system gets. Let's take a look at the following definition, which is given by Fran?ois Chollet in his book, Deep Learning with Python:
The definition quite aptly captures all of the necessary ingredients of DL and beautifully introduces the concept of treating data as a layered representation. So, a DL system, in a broad sense, breaks down the data into simple representations in a layered fashion, and to learn these representations, it often makes use of many layers (which is referred to as deep). We will now take a look at the big picture, which tells us how AI, ML, and DL are related to each other.