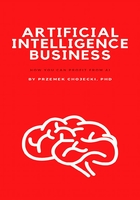
Artificial Intelligence Paradigm
Though it might seem like we’ve come a long way in the last ten years, which is true from a research perspective, the adoption of AI among corporations is still relatively low. According to some studies, only around 20% of companies are experimenting with AI. Most of the companies have only started to dabble with artificial intelligence.
This new era of information depends heavily on the knowledge, and we’re currently missing a lot of experts.
There’s a lot of fear and hype around AI, and it’s crucial to have as many people as possible to know about AI in order to understand what’s possible and what’s not. Only an educated AI-wise society will be able to adopt the technology fully. The goal of this book is to make it happen sooner than later.
At business level decision-makers, project managers and executives need basic knowledge, understanding of the machine learning paradigm, which would allow them to apply practical algorithms to real use cases, driving business, and growing sales.
On the other hand, trust is a crucial ingredient in any system. Thus explainable AI, being able to explain why these automation systems, machine learning architecture, act in this way, will allow us to understand how it’s going to affect all of us: co-workers, citizens, humans. For that purpose, we need to think about re-education when it comes to our organizations and implementing AI elements in schools from the very start of education.
AI will mostly enhance what we do on a daily basis. It’s not going to be full automation most of the time, but an augmentation of how we do certain tasks and how we work. This way of collaboration will also require rewiring of how we think of machines.
That’s why we also need to think about regulations. AI is the atomic energy of our times, and we can either use it to produce bombs or use it to produce energy. The standard paradigm of computing is based on ‘if-then’ loops. Coders give instructions to computers, supervising every single step of computing. Machine learning changed that completely. Coders don’t have to code every single step to make the computer work. They can just build a general architecture, like a specific neural network, and supply data in order to ‘train’ this architecture - that is, let the computer system self-tune as it sees fit by analysing data. This approach is more similar to teaching a child a particular task or introducing a junior coworker to a particular business process for the first time. And as it is the case with children, it often takes time for them to grasp the concept fully. It’s similar to machines. They need retraining and rebuilding parts of their architecture to excel at a given task.
Because of this shift of paradigm, it became possible to automate more tasks and business processes than ever before. You don’t have to program every single step of the process, predicting at each step what might happen and how to react to that. You can leave many of those details to algorithms, letting them see data and decide for themselves in each case how it should be solved.
Of course, that’s theory. In practice, things can get messy. AI is not a magic wand, and it doesn’t solve any problem you throw at it. You need good preparation to really benefit from artificial intelligence. This includes:
- Clearly describing what business process you want to automate or optimize.
- Defining what the output of the process is and how to distinguish between good or bad results. If that’s not possible, or if this is a continuous process without an end, then define mid-steps and mid-results that are anticipated.
- Defining what the input of the process is, that is what kind of data you take into account when looking for the output.
- Acquiring large datasets related to the business process. Cleaning it by removing unnecessary parts and organizing it in one place and in one format (e.g. .doc files stored on a cloud).
Having done this work, you’re ready to start hiring data scientists to build machine learning algorithms for you. Often this preliminary work will be revised and enhanced with new data and new insights, but you don’t have to worry about it at the start of the process. The crucial part for you, as an executive, is being clear about what business process exactly you want to tackle with AI.
Explanation of this new machine learning paradigm and how to apply it in business is the main reason for writing this book. I believe that understanding how data science teams work, how machine learning models are constructed, and what they need to perform well, is crucial to be competitive amid today’s technological revolution.
It is also crucial for legislators, politicians, and philosophers to understand how the machine learning community operates and what is possible with AI, to make legislations and laws working for everyone involved. There’s definitely too much hype regarding AI when it comes to how fast it will disrupt human jobs as we know them. And even though I believe that will eventually happen, what we should prepare for is the next 5-10-20 years of growing dependence on AI. Every job will be enhanced by artificial intelligence, rather than replaced by it, and we shouldn’t be scared of it. Examples in art and video games show that perfectly. We can learn a lot from the way machine learning systems look at our world and how they transpose it.
The most important thing is to stay open, embrace new technologies, and learn constantly. After all, what humans do best is adapt,