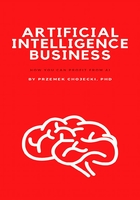
Solving maturity issues
Let’s now talk about how a company can transition to the next level, no matter where it starts. Building AI maturity is crucial in staying competitive in our digital world. Enterprises are challenged and beaten by technological startups because of a lack of innovation or a too-slow adaptation to changes. In this section, I discuss each level and potential challenges associated with it.
Awareness (1)
In order to ascend to the very first level, one should organize a workshop for the whole department about AI, machine learning, data science, and their potential use in real-world scenarios.
Potential challenges:
- educating staff of new ways of doing marketing,
- overcoming fear of AI and explaining it in simple terms to everyone.
From Awareness (1) to Active (2)
To progress past the first stage, one needs to connect the company’s data to the system able to analyse data. This way, the first generic algorithms can be put into action, and the user will get first visualisations and preliminary cleaning of data. This can be an external ready-to-use system built by another company, more experienced with AI. Depending on the context, an enterprise can start with:
- social media monitoring,
- competition monitoring,
- documents analysis,
- invoice analysis.
Self-service analytics becomes a significant factor in making decisions based on data, as they allow us to observe threats and opportunities for growth.
Moreover, one needs to form a team of data scientists or programmers who would operate daily with external systems and machine learning models. At this stage, it can be done by hiring external teams to complete the first pilots and have a sense of AI capabilities.
The whole department needs a workshop to get accustomed to the machine learning framework and thinking about data and processes with an AI in view.
Potential challenges:
- finding talented people within the organisation or hiring a new team to use a machine learning system; can be solved using an AI consultant agency.
- learning to use external platforms; getting accustomed to new software is often hard; people need time and workshops to overcome fears.
From Active (2) to Operational (3)
Data Science Lead is hired to manage the data science team and introduce new AI experts, build a practice, and form a vision for future products.
The company starts using a machine learning/analytics system to experiment with machine learning models and extract data.
The company goes beyond analytics into more advanced methods like:
- NLP for analysis of customers’ feedback,
- prediction of future customer behaviors,
- segmentation of customers into similarity groups using AI.
Potential challenges:
- attracting Data Science Lead is hard; one needs to spend usually a lot to attract top-notch talent and create an excellent environment for growth, which would be open and research-friendly.
From Operational (3) to Systemic (4)
At this stage, the AI team should work on their own algorithms, which then would be deployed within existing and new products.
Implementing a visual system for building models will allow for fast iteration, testing, and deployment.
There is an active communication channel between the AI team and the rest of the department.
Potential challenges:
- open communication channels between the AI team and the rest; the way to overcome it may be organizing regular 10 min stand-ups to ask questions
- expanding the AI team with machine learning engineers; hard to attract talent - if Data Science Lead is really good, he’ll be able to do it though.
From Systemic (4) to Transformational (5)
The whole department is educated in machine learning frameworks and AI pipeline through a series of workshops, which are run on a continuous basis. This will provide broad AI training to the whole team.
To each project/team, there is assigned an AI supervisor responsible for thinking about how AI can transform a given project or change it substantially.
The company leverages the in-house AI team with its own solutions as well as available platforms/services and open-source code (GitHub).
Decision-making is based on data and is enhanced with AI.
Potential challenges:
- explaining how real AI works - without overhyping it and showing weaknesses as well as strengths; it often takes time to do that part (workshops, seminars, one on one meetings).
- building a framework for what is being done internally and what externally when it comes to data and algorithms; it takes an understanding of what the goal of the company is, where lies the competitive advantage, and what will be the most important in 5 years.