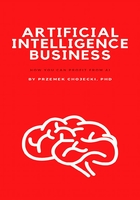
Research in Artificial Intelligence
The research community in Artificial Intelligence can be split into three divisions:
- machine learning community
- ethics and social community
- business community
Machine Learning community is concerned primarily with research questions related to building machine learning models: from architecture through data to implementations. PhD in computer science or STEM field is necessary to participate actively in it.
Ethics and social community focuses on social ramifications of doing AI research and applying it in practice: from legislations to important questions or limits on what should be the goal of AI research. People in this community often work in social departments of universities, think tanks, or public institutions.
Business community focuses on applying cutting-edge research to business problems. Those may include manufacturing, drug design, cybersecurity, video games, and others. Researchers here work mostly at research labs of large organisations. PhD is not necessary, but often an additional advantage when it comes to looking for a job in those.
If one wants to become a researcher in AI, the standard road is via university, doing a PhD in computer science, and then becoming an assistant professor or a research fellow. Thanks to recent changes in how research works, for example how Amazon, Facebook, Google and similar large tech companies are participating in doing research, it often happens that freshly mint PhDs go directly to one of tech giants’ research laboratories. It’s also possible that they do simultaneously PhD and work at one of those companies which is beneficial to each party: a company sponsors a PhD, the university is relieved from costs, a PhD student has a job and does something relevant to the industry.
PhD thesis itself is a monograph discussing and solving an open problem or some case of it, using novel methods in an already established problem or inventing new problems related to existing knowledge. Some topics are more in fashion at a particular time than others and this relates to interests of particular professors or interest of the market (where the money is). Often during the time of doing a PhD a student publishes a couple of papers, which then consists of the main body of a PhD thesis.
For a PhD student, the most important is finding a good advisor with access to interesting problems, funds, and a research group. Interesting problems will allow him to do meaningful research, funds will allow him to travel to conferences and spend money on infrastructure, the research group will be invaluable for research discussions.
Going to conferences is a great way to connect with fellow scientists. The most popular and most prestigious machine learning conference is NIPS, Neural Information Processing Systems’ annual meeting. The number of scientists applying with their papers to NIPS is growing by 30% each year, which also shows how lively is the machine learning community currently.
From the point of view of business that competes with academia for talent, the crucial aspect is creating a vibrant environment to do research in. Assigning free time to do any research is a good solution, but crucial is building a research group around a senior figure in the field. It was often the case that large organisations hired a professor from the machine learning department together with his PhD students as a way to start up a research community quickly. For example, this is what Uber did with poaching people from Carnegie Mellon’s robotics department.5
A big problem for established institutions like banks or insurers is presenting themselves in an appealing way to potential machine learning employees. Crucial here is understanding that what’s appealing to researches is being able to innovate, have freedom of thought, an atmosphere of openness, and hard problems at hand to solve. No one wants to be stuck with linear regressions all the time. It’s often better to pose too hard problems than too easy problems to attract talent (think Tesla or SpaceX).
Excellent examples of good problems are on Kaggle (www.kaggle.com), where companies run data science challenges for their business problems setting a prize for top entries. Often these competitions are attended by thousands of teams. One of the most famous ones was a competition run by Netflix6 to make better their recommendation algorithms. By putting the prize at $1M the competition attracted a lot of data scientists, put Netflix on a map of great tech companies to work at, and gave Netflix a lot of new research input relevant to their business operation.